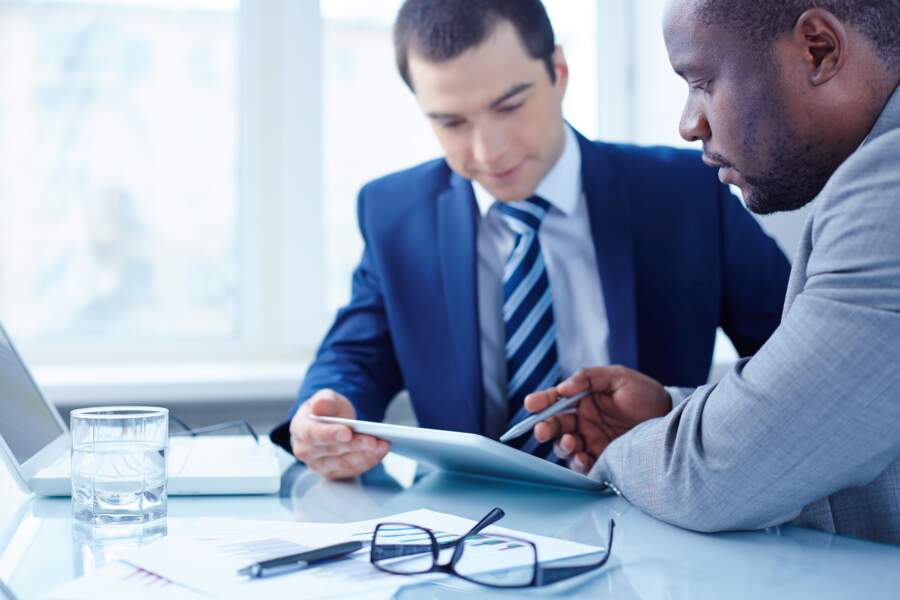
By Tim Tunnard, Senior Business Development Manager, Peacock Engineering
Organisations running and maintaining physical assets have to engage in a balancing act of maximising uptime, safety and longevity, against the need to keep control of maintenance costs, and perform maintenance in the most optimal way. Depending on the nature of the organisation and the assets involved, the balance point can be very different – for example, a power generation utility with grid capacity commitments will see maximising asset uptime and safety as critical. Conversely, a facilities management business running electrical services in a building may see controlling maintenance costs and SLA compliance as their priority.
In the past, lack of data on asset performance has meant that most asset managers have had to adopt one of two maintenance strategies:
1. Over-maintain – for organisations running critical infrastructure, or where public safety is involved, and assets must be kept running at all costs
2. Run-to-fail – where uptime is not critical, and cost control is paramount, this has traditionally been the most cost effective maintenance strategy
Now, however, the ability to capture data on asset performance and to then be able to process it immediately via Enterprise Asset Management systems (EAM) is enabling the possibility of a new maintenance strategy – Predictive Maintenance.
Predictive Maintenance
Predictive Maintenance relies on analysing data for an asset (or asset class), to establish over a time the likely failure point for that asset. Once an accurate picture of an asset’s performance is built up, a company can add algorithms which can automatically schedule maintenance at optimum points to avoid asset failure. To implement this successfully in an organisation, it is vital that all the data on asset performance is collected systematically (usually automatically), and it’s the right sort of data, and the best way of doing that is by using an EAM system.
Mobile data
Recent innovations in mobile asset management systems also now means maintenance crews can add data directly to the EAM at every visit and make or escalate decisions on the spot. This avoids any delays or inaccuracies with the data, which is vital preparation for implementing Predictive Maintenance.
The EAM asset data can be combined with data collected automatically from remote sensors, using Internet of Things technology (for example, SCADA sensors), to finesse the intervention points according to the parameters set – such as average temperature, read by a heat sensor, or the wear rate of a bearing, measured using a vibration sensor.
The same sensors can be used to trigger alarms for immediate intervention, via the EAM, according to the conditions set. But users of Predictive Maintenance generally find that sensor-based monitoring reduces the requirement for manual intervention, by screening out false alarms, making data-based decisions about the state of the asset, and reducing maintenance visits.
Less maintenance, more uptime
Predictive Maintenance is all about being able to strike the right balance in an asset maintenance strategy. It can significantly reduce maintenance on each asset, and result in greater uptime. Customers using Predictive Maintenance often report startling results – for example, one global car manufacturer that used Maximo saw its manufacturing downtime slashed by 50%.
The EAM system then monitors and leverages the company’s maintenance benchmark KPIs such as MTBF (meantime between failure) and MTTF (meantime to failure), which users can use to predict the likely best time to perform proactive maintenance on the asset. The EAM system can then automatically schedule the maintenance with the appropriate work crew.
Reducing asset failure
In the event of an asset failure, the EAM data will conduct a Root Cause Analysis, and use data collected about the failure, to improve the modelling for that asset. This helps minimise future failures for the individual asset, and any similar ones.
It’s important to note though that equipment failure is an important part of the AI learning process, and that with the complexity of variables affecting each asset (for example: weather, fuel quality, frequency of use), it can take some time for algorithms to deliver the accuracy that Predictive Maintenance requires.
In particular industries safety and legal compliance are of paramount concern. Some of these industries may also be facing multiple challenges – e.g. falling prices, increased competition, heavy regulation, and underperformance penalties. In these situations, it is critical that businesses take advantage of new technologies wherever possible. Using AI based Predictive Maintenance is one way to square the circle – enabling them to increase reliability of assets, while simultaneously reducing maintenance costs.
The increased reliability that Predictive Maintenance brings is also helpful to businesses dealing directly with the public. Reducing the chances of catastrophic failures and getting early warnings of possible outages or otherissues, avoids the potential for financial and reputational damage which can be caused by such failures.
Although Predictive Maintenance is still in its infancy, the potential is clearly huge. Collecting the right data is a key first step. along with an Enterprise Asset Management system – vital for collating that data to then be able to use for Predictive Maintenance. Together they make an effective asset management and maintenance strategy that means less maintenance spend and greater productivity.