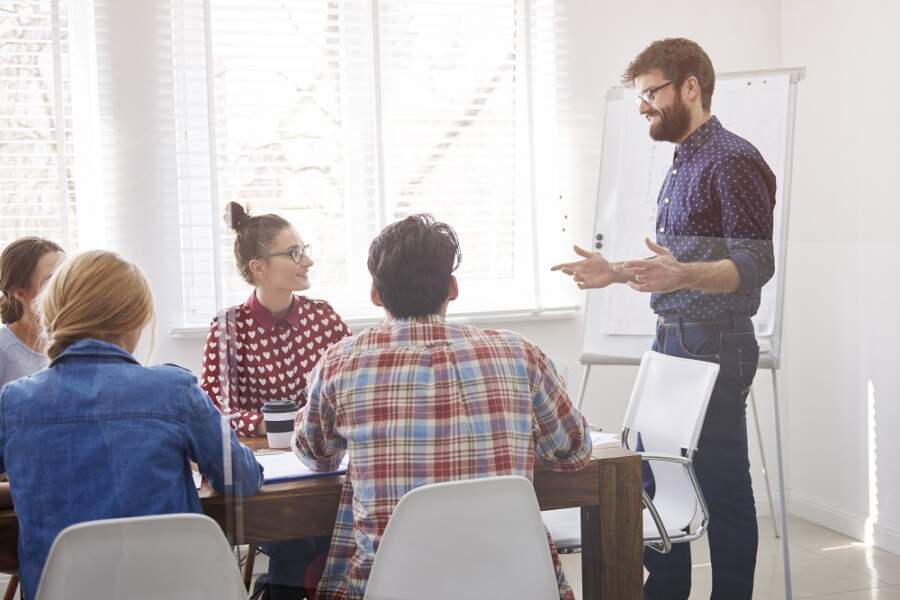
Hunting the longtail: Five steps to solve unplanned downtime
How do organisations get to the root of annoying maintenance problems and tackle the troublesome reality of a loss in productivity?
There are some unsubstantiated stats doing the rounds at the moment, on the cost of unplanned downtime for manufacturers. These stats are putting average losses at over £1 trillion but while this is difficult to really measure, all organisations will have their own pain points where downtime is costing the business. What the actual cost is will vary but what is clear is that in this age of the “Digital Thread” – IoT sensors, advanced cloud-based infrastructures, AI/ML-driven automation and analytics, and a feedback loop to product engineering, we have the tools to try and solve problems that lead to downtime.
Below are five steps to help track down persistent maintenance issues:
Measure Those Assets
To really get to grips with machine performance and to try and understand error rates, it is essential that asset data is captured and analysed. One way is to leverage the concept of a “Digital Twin” (the expected performance of an asset in the field) to its actual performance via IoT devices and sensors installed on the asset itself – this year IoT is expected to see significant growth, according to Verdict research. The global IoT market will be worth a whopping $650bn by the end of this year, as organisations recognise the value of machine and device intelligence in real time.
“Advances to the industrial internet will be accelerated through increased network agility, integrated AI and the capacity to deploy, automate, orchestrate and secure diverse use cases at hyperscale,” says an Ericsson report The Future of IoT. “The potential is not just in enabling billions of devices simultaneously but leveraging the huge volumes of actionable data which can automate diverse business processes.” By comparing how an asset should be performing to how it is actually performing, we gain insights into what that assets maintenance needs are and provide critical data to product engineering to continuously improve the asset design.
This is key to optimising not just service capabilities but to extend the asset uptime, service life, and in the process increase customer productivity.
Unify The Data
Gartner suggests that poor data quality costs organisations $12.9 million every year adding that, over the long term, it “increases the complexity of data ecosystems and leads to poor decision making.” A big driver of poor data quality is errors in the transcription of data and having multiple different procedures for data collection. Automated IOT data collection reduces the opportunity for manually inserting errors and standardizes the process for data collection simplifying data aggregation and utilization.
Another issue is data silos. Organisations need to unify and standardize their data to provide a comprehensive picture of the business, assets and customers. This will help leaders make informed decisions on end-of-life products or consistently under-performing products and then deliver ideas to customers on how to improve uptime and productivity.
It also enables organisations to plan, ensuring optimisation and profitability through a complete and accurate picture of customer contracts, renewals and upgrades. As Deloitte suggested in its report Next Generation Customer Service: The Future of Field Service, to transform to next generation field service, businesses need a 360-degree view of both customers and their assets.
Predict Failures
AI/ML-enabled analytics, leveraging a Digital Twin and the Digital Thread (from product design, through engineering, manufacture, installation, and maintenance) can deliver predictive maintenance capabilities, to identify potential problems with machines and devices before there is a failure. As organisations move towards more outcome-based arrangements with customers, having SLAs that guarantee uptime, for example, will demand real-time analytics capabilities and rapid execution of maintenance delivery when a problem is indicated.
Asset intelligence is central to prediction. Comparing actual asset performance data to the Digital Twin for that asset, leveraging AI automation and analytics to identify potential issues, finding and ordering components or parts, and despatching maintenance teams to deliver services before a failure occurs. As well as extending the life of existing assets, this removes the fear of unplanned downtime and identifies those potential long tail service issues before they become long tails.
All of this drives increased customer satisfaction as well as the opportunity to grow contract revenue for manufacturers and value for customers.
Optimise Maintenance Teams
According to a report on transforming field service with emerging technologies, customer-centricity and personalization (68%); face-to-face or in-person field service appointments (55%); and leveraging service technicians as brand representatives or salespeople (51%) will become more important over the next three years. The point is that the service team is changing, and access to accurate and timely data is making it all possible.
Using the Digital Thread, accessing asset data, predictive analytics and optimised supply chain deliveries and inventory, organisations can reduce costs, and wasted journey times for engineers, through optimised service work. By reduce truck rolls, organisations can save time and money as well as improve service efficiency for customers.
Engage Product Design Teams
With any longtail service problem – traditionally this has been down to poor product or machine design leading to persistent maintenance issues – organisations now have the intelligence to understand the condition of the asset in the field, find the areas that require reengineering, and react proactively rather than reactively.
Assets can deliver qualitative data to organisations which should be fed back to engineering, design, and development teams to solve recurring product issues. With unified data, real-time asset data and AI automation and analytics, this should close the loop between product lifecycle management and service lifecycle management.
As a PwC report on The importance of the circular economy in manufacturing claimed, bringing the concept of the circular economy to life within the manufacturing value chain “involves substantial changes in core production and supply chain processes,” which would also require a “reverse logistics process to get the used products back into the cycle.”
This makes sense. By focusing on the entire lifecycle of a product (design, engineering, manufacture, and service) and not just its recyclability, manufacturers can start to shift their thinking towards longer product life and efficiencies in service provision, putting an end to the longtail service issues and getting to the root of unplanned downtime problems.
Joseph Kenny is Vice President, Global Customer Transformation at ServiceMax.